$E(T_i) = 1 + frac{1}{i-1}sum_{j=1}^{i-1}E(T_j)$ [on hold]
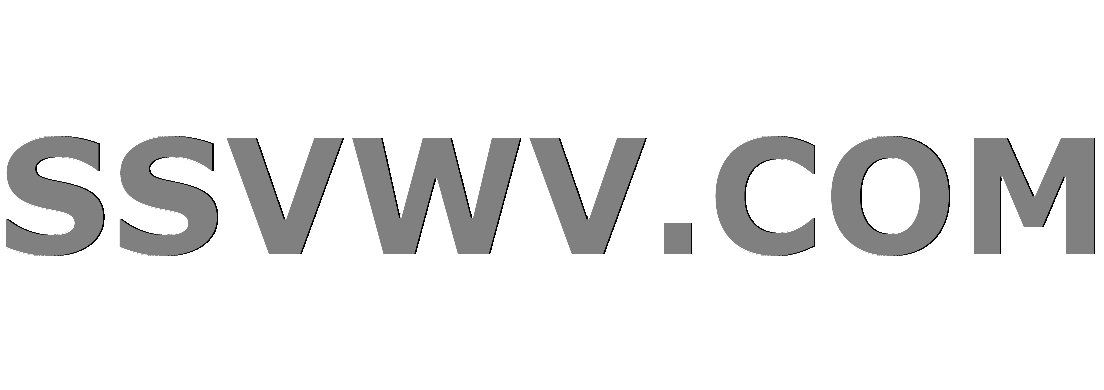
Multi tool use
Consider a markov chain for which $P_{11}=1$ and
$$P_{ij}= frac{1}{i-1}, spacespacespace j=1,dots,i-1, i>1$$
[$P=((P_{ij}))$ is transition matrix.] Let $T_i$ denote the number of transitions to go state $i$ to state $1$. A recursive formula for $E(T_i)$ is:
$$E(T_i) = 1 + frac{1}{i-1}sum_{j=1}^{i-1}E(T_j) tag{1}$$
I could not understand how $(1)$ can be obtained. Thanks for any help.
probability probability-theory stochastic-processes markov-chains
put on hold as unclear what you're asking by Did, clathratus, amWhy, Adrian Keister, KReiser Jan 1 at 2:28
Please clarify your specific problem or add additional details to highlight exactly what you need. As it's currently written, it’s hard to tell exactly what you're asking. See the How to Ask page for help clarifying this question. If this question can be reworded to fit the rules in the help center, please edit the question.
add a comment |
Consider a markov chain for which $P_{11}=1$ and
$$P_{ij}= frac{1}{i-1}, spacespacespace j=1,dots,i-1, i>1$$
[$P=((P_{ij}))$ is transition matrix.] Let $T_i$ denote the number of transitions to go state $i$ to state $1$. A recursive formula for $E(T_i)$ is:
$$E(T_i) = 1 + frac{1}{i-1}sum_{j=1}^{i-1}E(T_j) tag{1}$$
I could not understand how $(1)$ can be obtained. Thanks for any help.
probability probability-theory stochastic-processes markov-chains
put on hold as unclear what you're asking by Did, clathratus, amWhy, Adrian Keister, KReiser Jan 1 at 2:28
Please clarify your specific problem or add additional details to highlight exactly what you need. As it's currently written, it’s hard to tell exactly what you're asking. See the How to Ask page for help clarifying this question. If this question can be reworded to fit the rules in the help center, please edit the question.
Notice that $mathbb{E}(T_i) = mathbb{E}_i(H_1)$ where $H_1$ is the hitting time of the state $1$, and apply the formula for the expectation of hitting times found here for instance. You can also check out Theorem 1.3.5 from the book Markov Chains by J.R. Norris.
– Michh
Dec 28 '18 at 0:32
"I could not understand how (1) can be obtained" ?? This is the most basic one-step Markov property of the process.
– Did
Dec 31 '18 at 20:13
add a comment |
Consider a markov chain for which $P_{11}=1$ and
$$P_{ij}= frac{1}{i-1}, spacespacespace j=1,dots,i-1, i>1$$
[$P=((P_{ij}))$ is transition matrix.] Let $T_i$ denote the number of transitions to go state $i$ to state $1$. A recursive formula for $E(T_i)$ is:
$$E(T_i) = 1 + frac{1}{i-1}sum_{j=1}^{i-1}E(T_j) tag{1}$$
I could not understand how $(1)$ can be obtained. Thanks for any help.
probability probability-theory stochastic-processes markov-chains
Consider a markov chain for which $P_{11}=1$ and
$$P_{ij}= frac{1}{i-1}, spacespacespace j=1,dots,i-1, i>1$$
[$P=((P_{ij}))$ is transition matrix.] Let $T_i$ denote the number of transitions to go state $i$ to state $1$. A recursive formula for $E(T_i)$ is:
$$E(T_i) = 1 + frac{1}{i-1}sum_{j=1}^{i-1}E(T_j) tag{1}$$
I could not understand how $(1)$ can be obtained. Thanks for any help.
probability probability-theory stochastic-processes markov-chains
probability probability-theory stochastic-processes markov-chains
asked Dec 27 '18 at 13:15


Stat_prob_001
297113
297113
put on hold as unclear what you're asking by Did, clathratus, amWhy, Adrian Keister, KReiser Jan 1 at 2:28
Please clarify your specific problem or add additional details to highlight exactly what you need. As it's currently written, it’s hard to tell exactly what you're asking. See the How to Ask page for help clarifying this question. If this question can be reworded to fit the rules in the help center, please edit the question.
put on hold as unclear what you're asking by Did, clathratus, amWhy, Adrian Keister, KReiser Jan 1 at 2:28
Please clarify your specific problem or add additional details to highlight exactly what you need. As it's currently written, it’s hard to tell exactly what you're asking. See the How to Ask page for help clarifying this question. If this question can be reworded to fit the rules in the help center, please edit the question.
Notice that $mathbb{E}(T_i) = mathbb{E}_i(H_1)$ where $H_1$ is the hitting time of the state $1$, and apply the formula for the expectation of hitting times found here for instance. You can also check out Theorem 1.3.5 from the book Markov Chains by J.R. Norris.
– Michh
Dec 28 '18 at 0:32
"I could not understand how (1) can be obtained" ?? This is the most basic one-step Markov property of the process.
– Did
Dec 31 '18 at 20:13
add a comment |
Notice that $mathbb{E}(T_i) = mathbb{E}_i(H_1)$ where $H_1$ is the hitting time of the state $1$, and apply the formula for the expectation of hitting times found here for instance. You can also check out Theorem 1.3.5 from the book Markov Chains by J.R. Norris.
– Michh
Dec 28 '18 at 0:32
"I could not understand how (1) can be obtained" ?? This is the most basic one-step Markov property of the process.
– Did
Dec 31 '18 at 20:13
Notice that $mathbb{E}(T_i) = mathbb{E}_i(H_1)$ where $H_1$ is the hitting time of the state $1$, and apply the formula for the expectation of hitting times found here for instance. You can also check out Theorem 1.3.5 from the book Markov Chains by J.R. Norris.
– Michh
Dec 28 '18 at 0:32
Notice that $mathbb{E}(T_i) = mathbb{E}_i(H_1)$ where $H_1$ is the hitting time of the state $1$, and apply the formula for the expectation of hitting times found here for instance. You can also check out Theorem 1.3.5 from the book Markov Chains by J.R. Norris.
– Michh
Dec 28 '18 at 0:32
"I could not understand how (1) can be obtained" ?? This is the most basic one-step Markov property of the process.
– Did
Dec 31 '18 at 20:13
"I could not understand how (1) can be obtained" ?? This is the most basic one-step Markov property of the process.
– Did
Dec 31 '18 at 20:13
add a comment |
0
active
oldest
votes
0
active
oldest
votes
0
active
oldest
votes
active
oldest
votes
active
oldest
votes
2OBvDn ROuLDGcfM31PbQ
Notice that $mathbb{E}(T_i) = mathbb{E}_i(H_1)$ where $H_1$ is the hitting time of the state $1$, and apply the formula for the expectation of hitting times found here for instance. You can also check out Theorem 1.3.5 from the book Markov Chains by J.R. Norris.
– Michh
Dec 28 '18 at 0:32
"I could not understand how (1) can be obtained" ?? This is the most basic one-step Markov property of the process.
– Did
Dec 31 '18 at 20:13