Can discrete time series be non-stationary?
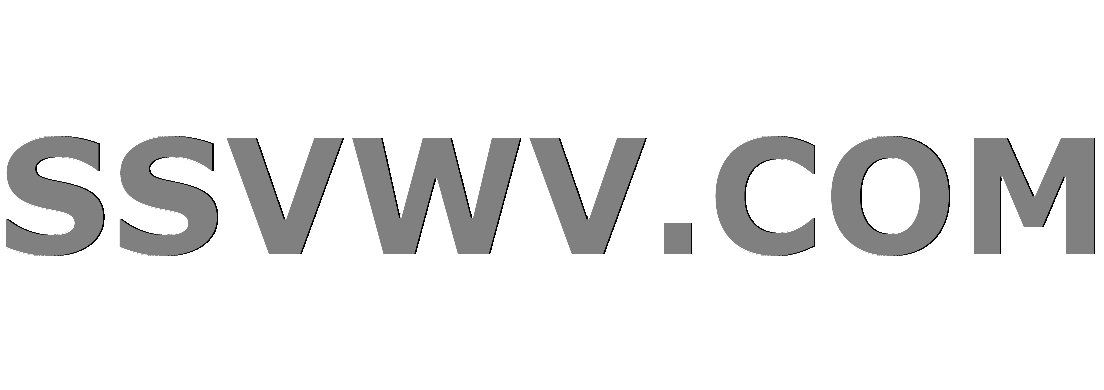
Multi tool use
$begingroup$
We all know about time series that grow over time, but it seems like we only ever see continuous values such as the plot shown below.
But is there such thing as a time series that might start out with balanced mix of class 0 and class 1, but eventually turn into mostly class 1's?
time-series stationarity
$endgroup$
add a comment |
$begingroup$
We all know about time series that grow over time, but it seems like we only ever see continuous values such as the plot shown below.
But is there such thing as a time series that might start out with balanced mix of class 0 and class 1, but eventually turn into mostly class 1's?
time-series stationarity
$endgroup$
$begingroup$
You are confused about the meaning of "continuous". Your data is not continuous, it is discrete: the algorithm that draws a line is filling the gaps and displays a continuous line, but the underlying data is discrete. Continuous data exists only in our minds. The world is discrete. Even if the gaps between data points is 1e-100 second.
$endgroup$
– PatrickT
Jan 26 at 10:51
add a comment |
$begingroup$
We all know about time series that grow over time, but it seems like we only ever see continuous values such as the plot shown below.
But is there such thing as a time series that might start out with balanced mix of class 0 and class 1, but eventually turn into mostly class 1's?
time-series stationarity
$endgroup$
We all know about time series that grow over time, but it seems like we only ever see continuous values such as the plot shown below.
But is there such thing as a time series that might start out with balanced mix of class 0 and class 1, but eventually turn into mostly class 1's?
time-series stationarity
time-series stationarity
asked Jan 26 at 3:57
Linas KleizaLinas Kleiza
394
394
$begingroup$
You are confused about the meaning of "continuous". Your data is not continuous, it is discrete: the algorithm that draws a line is filling the gaps and displays a continuous line, but the underlying data is discrete. Continuous data exists only in our minds. The world is discrete. Even if the gaps between data points is 1e-100 second.
$endgroup$
– PatrickT
Jan 26 at 10:51
add a comment |
$begingroup$
You are confused about the meaning of "continuous". Your data is not continuous, it is discrete: the algorithm that draws a line is filling the gaps and displays a continuous line, but the underlying data is discrete. Continuous data exists only in our minds. The world is discrete. Even if the gaps between data points is 1e-100 second.
$endgroup$
– PatrickT
Jan 26 at 10:51
$begingroup$
You are confused about the meaning of "continuous". Your data is not continuous, it is discrete: the algorithm that draws a line is filling the gaps and displays a continuous line, but the underlying data is discrete. Continuous data exists only in our minds. The world is discrete. Even if the gaps between data points is 1e-100 second.
$endgroup$
– PatrickT
Jan 26 at 10:51
$begingroup$
You are confused about the meaning of "continuous". Your data is not continuous, it is discrete: the algorithm that draws a line is filling the gaps and displays a continuous line, but the underlying data is discrete. Continuous data exists only in our minds. The world is discrete. Even if the gaps between data points is 1e-100 second.
$endgroup$
– PatrickT
Jan 26 at 10:51
add a comment |
2 Answers
2
active
oldest
votes
$begingroup$
Per the title, certainly discrete series can be nonstationary.
The total count of events in a Poisson process is one example - let $N_t$ be the number of events by time $t$ and consider the time series formed by looking at $N_t$ at integer times: $N_1$, $N_2$, $N_3$,...
We have that $E(N_t)=lambda t$, $text{Var}(N_t)=lambda t$; so the above time series is not even weakly stationary.
But is there such thing as a time series that might start out with balanced mix of class 0 and class 1, but eventually turn into mostly class 1's?
Sure, you could readily construct a binary time series process that was non-stationary.
Let's make an example. Let $Y_t$ be the random binary value at time $t$ ($t=1,2,...$). Let $S_t$ bet the number of $1$'s observed up to time $t$; i.e. $S_0=0,S_t=sum_{i=1}^t Y_i,,: t = 1,2,...$.
Now make $p_{t+1}=P(Y_{t+1}=1|S_t)=frac{1}{1+exp(-alpha S_t)}$ for some $alpha>0$.
So $p_1=0.5$. It will remain at $0.5$ until you observe a $1$, at which point $p$ increases. e.g. if $alpha=0.3$, then after the first $1$ is observed, $p$ increases to about $0.574$ whereas if $alpha=0.1$, $p$ only increases to about $0.525$.
(There are many other ways to make nonstationary binary series)
Here's an example using the above scheme with $alpha=0.005$, for the first 1000 observations. You can see that it starts out with a similar density of 0 and 1 values but the 1-strip rapidly starts to get heavier and the 0-strip gets lighter and lighter, until near the end the 0's have become quite rare.
The conditional probability of a 1 for the last observation ($y_{1000}$) was about 0.979.
$endgroup$
$begingroup$
Thanks for the example. But for continuous non-stationary time series you can take the differences to make it stationary. Is there an analogous procedure for non-stationary discrete time series?
$endgroup$
– Linas Kleiza
Jan 26 at 7:04
2
$begingroup$
Not in general, no; it would work with the Poisson example I raised, but does not work for the binary one. (For that matter, it's not always the case that differencing results in stationarity with continuous series. For some very particular sets of assumptions differencing produces stationarity, but not all. You might like to consider a series that's conditionally beta (i.e. continuous) with a similar setup to the binary example and see that differencing that series wouldn't result in even weak stationarity.)
$endgroup$
– Glen_b♦
Jan 26 at 7:16
add a comment |
$begingroup$
Yes, they can: For example, take any non-stationary time series and then take its values only at discrete intervals. That will (almost always) give you a non-stationary discrete time series.
$endgroup$
add a comment |
Your Answer
StackExchange.ifUsing("editor", function () {
return StackExchange.using("mathjaxEditing", function () {
StackExchange.MarkdownEditor.creationCallbacks.add(function (editor, postfix) {
StackExchange.mathjaxEditing.prepareWmdForMathJax(editor, postfix, [["$", "$"], ["\\(","\\)"]]);
});
});
}, "mathjax-editing");
StackExchange.ready(function() {
var channelOptions = {
tags: "".split(" "),
id: "65"
};
initTagRenderer("".split(" "), "".split(" "), channelOptions);
StackExchange.using("externalEditor", function() {
// Have to fire editor after snippets, if snippets enabled
if (StackExchange.settings.snippets.snippetsEnabled) {
StackExchange.using("snippets", function() {
createEditor();
});
}
else {
createEditor();
}
});
function createEditor() {
StackExchange.prepareEditor({
heartbeatType: 'answer',
autoActivateHeartbeat: false,
convertImagesToLinks: false,
noModals: true,
showLowRepImageUploadWarning: true,
reputationToPostImages: null,
bindNavPrevention: true,
postfix: "",
imageUploader: {
brandingHtml: "Powered by u003ca class="icon-imgur-white" href="https://imgur.com/"u003eu003c/au003e",
contentPolicyHtml: "User contributions licensed under u003ca href="https://creativecommons.org/licenses/by-sa/3.0/"u003ecc by-sa 3.0 with attribution requiredu003c/au003e u003ca href="https://stackoverflow.com/legal/content-policy"u003e(content policy)u003c/au003e",
allowUrls: true
},
onDemand: true,
discardSelector: ".discard-answer"
,immediatelyShowMarkdownHelp:true
});
}
});
Sign up or log in
StackExchange.ready(function () {
StackExchange.helpers.onClickDraftSave('#login-link');
});
Sign up using Google
Sign up using Facebook
Sign up using Email and Password
Post as a guest
Required, but never shown
StackExchange.ready(
function () {
StackExchange.openid.initPostLogin('.new-post-login', 'https%3a%2f%2fstats.stackexchange.com%2fquestions%2f389230%2fcan-discrete-time-series-be-non-stationary%23new-answer', 'question_page');
}
);
Post as a guest
Required, but never shown
2 Answers
2
active
oldest
votes
2 Answers
2
active
oldest
votes
active
oldest
votes
active
oldest
votes
$begingroup$
Per the title, certainly discrete series can be nonstationary.
The total count of events in a Poisson process is one example - let $N_t$ be the number of events by time $t$ and consider the time series formed by looking at $N_t$ at integer times: $N_1$, $N_2$, $N_3$,...
We have that $E(N_t)=lambda t$, $text{Var}(N_t)=lambda t$; so the above time series is not even weakly stationary.
But is there such thing as a time series that might start out with balanced mix of class 0 and class 1, but eventually turn into mostly class 1's?
Sure, you could readily construct a binary time series process that was non-stationary.
Let's make an example. Let $Y_t$ be the random binary value at time $t$ ($t=1,2,...$). Let $S_t$ bet the number of $1$'s observed up to time $t$; i.e. $S_0=0,S_t=sum_{i=1}^t Y_i,,: t = 1,2,...$.
Now make $p_{t+1}=P(Y_{t+1}=1|S_t)=frac{1}{1+exp(-alpha S_t)}$ for some $alpha>0$.
So $p_1=0.5$. It will remain at $0.5$ until you observe a $1$, at which point $p$ increases. e.g. if $alpha=0.3$, then after the first $1$ is observed, $p$ increases to about $0.574$ whereas if $alpha=0.1$, $p$ only increases to about $0.525$.
(There are many other ways to make nonstationary binary series)
Here's an example using the above scheme with $alpha=0.005$, for the first 1000 observations. You can see that it starts out with a similar density of 0 and 1 values but the 1-strip rapidly starts to get heavier and the 0-strip gets lighter and lighter, until near the end the 0's have become quite rare.
The conditional probability of a 1 for the last observation ($y_{1000}$) was about 0.979.
$endgroup$
$begingroup$
Thanks for the example. But for continuous non-stationary time series you can take the differences to make it stationary. Is there an analogous procedure for non-stationary discrete time series?
$endgroup$
– Linas Kleiza
Jan 26 at 7:04
2
$begingroup$
Not in general, no; it would work with the Poisson example I raised, but does not work for the binary one. (For that matter, it's not always the case that differencing results in stationarity with continuous series. For some very particular sets of assumptions differencing produces stationarity, but not all. You might like to consider a series that's conditionally beta (i.e. continuous) with a similar setup to the binary example and see that differencing that series wouldn't result in even weak stationarity.)
$endgroup$
– Glen_b♦
Jan 26 at 7:16
add a comment |
$begingroup$
Per the title, certainly discrete series can be nonstationary.
The total count of events in a Poisson process is one example - let $N_t$ be the number of events by time $t$ and consider the time series formed by looking at $N_t$ at integer times: $N_1$, $N_2$, $N_3$,...
We have that $E(N_t)=lambda t$, $text{Var}(N_t)=lambda t$; so the above time series is not even weakly stationary.
But is there such thing as a time series that might start out with balanced mix of class 0 and class 1, but eventually turn into mostly class 1's?
Sure, you could readily construct a binary time series process that was non-stationary.
Let's make an example. Let $Y_t$ be the random binary value at time $t$ ($t=1,2,...$). Let $S_t$ bet the number of $1$'s observed up to time $t$; i.e. $S_0=0,S_t=sum_{i=1}^t Y_i,,: t = 1,2,...$.
Now make $p_{t+1}=P(Y_{t+1}=1|S_t)=frac{1}{1+exp(-alpha S_t)}$ for some $alpha>0$.
So $p_1=0.5$. It will remain at $0.5$ until you observe a $1$, at which point $p$ increases. e.g. if $alpha=0.3$, then after the first $1$ is observed, $p$ increases to about $0.574$ whereas if $alpha=0.1$, $p$ only increases to about $0.525$.
(There are many other ways to make nonstationary binary series)
Here's an example using the above scheme with $alpha=0.005$, for the first 1000 observations. You can see that it starts out with a similar density of 0 and 1 values but the 1-strip rapidly starts to get heavier and the 0-strip gets lighter and lighter, until near the end the 0's have become quite rare.
The conditional probability of a 1 for the last observation ($y_{1000}$) was about 0.979.
$endgroup$
$begingroup$
Thanks for the example. But for continuous non-stationary time series you can take the differences to make it stationary. Is there an analogous procedure for non-stationary discrete time series?
$endgroup$
– Linas Kleiza
Jan 26 at 7:04
2
$begingroup$
Not in general, no; it would work with the Poisson example I raised, but does not work for the binary one. (For that matter, it's not always the case that differencing results in stationarity with continuous series. For some very particular sets of assumptions differencing produces stationarity, but not all. You might like to consider a series that's conditionally beta (i.e. continuous) with a similar setup to the binary example and see that differencing that series wouldn't result in even weak stationarity.)
$endgroup$
– Glen_b♦
Jan 26 at 7:16
add a comment |
$begingroup$
Per the title, certainly discrete series can be nonstationary.
The total count of events in a Poisson process is one example - let $N_t$ be the number of events by time $t$ and consider the time series formed by looking at $N_t$ at integer times: $N_1$, $N_2$, $N_3$,...
We have that $E(N_t)=lambda t$, $text{Var}(N_t)=lambda t$; so the above time series is not even weakly stationary.
But is there such thing as a time series that might start out with balanced mix of class 0 and class 1, but eventually turn into mostly class 1's?
Sure, you could readily construct a binary time series process that was non-stationary.
Let's make an example. Let $Y_t$ be the random binary value at time $t$ ($t=1,2,...$). Let $S_t$ bet the number of $1$'s observed up to time $t$; i.e. $S_0=0,S_t=sum_{i=1}^t Y_i,,: t = 1,2,...$.
Now make $p_{t+1}=P(Y_{t+1}=1|S_t)=frac{1}{1+exp(-alpha S_t)}$ for some $alpha>0$.
So $p_1=0.5$. It will remain at $0.5$ until you observe a $1$, at which point $p$ increases. e.g. if $alpha=0.3$, then after the first $1$ is observed, $p$ increases to about $0.574$ whereas if $alpha=0.1$, $p$ only increases to about $0.525$.
(There are many other ways to make nonstationary binary series)
Here's an example using the above scheme with $alpha=0.005$, for the first 1000 observations. You can see that it starts out with a similar density of 0 and 1 values but the 1-strip rapidly starts to get heavier and the 0-strip gets lighter and lighter, until near the end the 0's have become quite rare.
The conditional probability of a 1 for the last observation ($y_{1000}$) was about 0.979.
$endgroup$
Per the title, certainly discrete series can be nonstationary.
The total count of events in a Poisson process is one example - let $N_t$ be the number of events by time $t$ and consider the time series formed by looking at $N_t$ at integer times: $N_1$, $N_2$, $N_3$,...
We have that $E(N_t)=lambda t$, $text{Var}(N_t)=lambda t$; so the above time series is not even weakly stationary.
But is there such thing as a time series that might start out with balanced mix of class 0 and class 1, but eventually turn into mostly class 1's?
Sure, you could readily construct a binary time series process that was non-stationary.
Let's make an example. Let $Y_t$ be the random binary value at time $t$ ($t=1,2,...$). Let $S_t$ bet the number of $1$'s observed up to time $t$; i.e. $S_0=0,S_t=sum_{i=1}^t Y_i,,: t = 1,2,...$.
Now make $p_{t+1}=P(Y_{t+1}=1|S_t)=frac{1}{1+exp(-alpha S_t)}$ for some $alpha>0$.
So $p_1=0.5$. It will remain at $0.5$ until you observe a $1$, at which point $p$ increases. e.g. if $alpha=0.3$, then after the first $1$ is observed, $p$ increases to about $0.574$ whereas if $alpha=0.1$, $p$ only increases to about $0.525$.
(There are many other ways to make nonstationary binary series)
Here's an example using the above scheme with $alpha=0.005$, for the first 1000 observations. You can see that it starts out with a similar density of 0 and 1 values but the 1-strip rapidly starts to get heavier and the 0-strip gets lighter and lighter, until near the end the 0's have become quite rare.
The conditional probability of a 1 for the last observation ($y_{1000}$) was about 0.979.
edited Jan 26 at 8:25
answered Jan 26 at 4:48


Glen_b♦Glen_b
213k22410760
213k22410760
$begingroup$
Thanks for the example. But for continuous non-stationary time series you can take the differences to make it stationary. Is there an analogous procedure for non-stationary discrete time series?
$endgroup$
– Linas Kleiza
Jan 26 at 7:04
2
$begingroup$
Not in general, no; it would work with the Poisson example I raised, but does not work for the binary one. (For that matter, it's not always the case that differencing results in stationarity with continuous series. For some very particular sets of assumptions differencing produces stationarity, but not all. You might like to consider a series that's conditionally beta (i.e. continuous) with a similar setup to the binary example and see that differencing that series wouldn't result in even weak stationarity.)
$endgroup$
– Glen_b♦
Jan 26 at 7:16
add a comment |
$begingroup$
Thanks for the example. But for continuous non-stationary time series you can take the differences to make it stationary. Is there an analogous procedure for non-stationary discrete time series?
$endgroup$
– Linas Kleiza
Jan 26 at 7:04
2
$begingroup$
Not in general, no; it would work with the Poisson example I raised, but does not work for the binary one. (For that matter, it's not always the case that differencing results in stationarity with continuous series. For some very particular sets of assumptions differencing produces stationarity, but not all. You might like to consider a series that's conditionally beta (i.e. continuous) with a similar setup to the binary example and see that differencing that series wouldn't result in even weak stationarity.)
$endgroup$
– Glen_b♦
Jan 26 at 7:16
$begingroup$
Thanks for the example. But for continuous non-stationary time series you can take the differences to make it stationary. Is there an analogous procedure for non-stationary discrete time series?
$endgroup$
– Linas Kleiza
Jan 26 at 7:04
$begingroup$
Thanks for the example. But for continuous non-stationary time series you can take the differences to make it stationary. Is there an analogous procedure for non-stationary discrete time series?
$endgroup$
– Linas Kleiza
Jan 26 at 7:04
2
2
$begingroup$
Not in general, no; it would work with the Poisson example I raised, but does not work for the binary one. (For that matter, it's not always the case that differencing results in stationarity with continuous series. For some very particular sets of assumptions differencing produces stationarity, but not all. You might like to consider a series that's conditionally beta (i.e. continuous) with a similar setup to the binary example and see that differencing that series wouldn't result in even weak stationarity.)
$endgroup$
– Glen_b♦
Jan 26 at 7:16
$begingroup$
Not in general, no; it would work with the Poisson example I raised, but does not work for the binary one. (For that matter, it's not always the case that differencing results in stationarity with continuous series. For some very particular sets of assumptions differencing produces stationarity, but not all. You might like to consider a series that's conditionally beta (i.e. continuous) with a similar setup to the binary example and see that differencing that series wouldn't result in even weak stationarity.)
$endgroup$
– Glen_b♦
Jan 26 at 7:16
add a comment |
$begingroup$
Yes, they can: For example, take any non-stationary time series and then take its values only at discrete intervals. That will (almost always) give you a non-stationary discrete time series.
$endgroup$
add a comment |
$begingroup$
Yes, they can: For example, take any non-stationary time series and then take its values only at discrete intervals. That will (almost always) give you a non-stationary discrete time series.
$endgroup$
add a comment |
$begingroup$
Yes, they can: For example, take any non-stationary time series and then take its values only at discrete intervals. That will (almost always) give you a non-stationary discrete time series.
$endgroup$
Yes, they can: For example, take any non-stationary time series and then take its values only at discrete intervals. That will (almost always) give you a non-stationary discrete time series.
answered Jan 26 at 9:24


BenBen
25.8k227121
25.8k227121
add a comment |
add a comment |
Thanks for contributing an answer to Cross Validated!
- Please be sure to answer the question. Provide details and share your research!
But avoid …
- Asking for help, clarification, or responding to other answers.
- Making statements based on opinion; back them up with references or personal experience.
Use MathJax to format equations. MathJax reference.
To learn more, see our tips on writing great answers.
Sign up or log in
StackExchange.ready(function () {
StackExchange.helpers.onClickDraftSave('#login-link');
});
Sign up using Google
Sign up using Facebook
Sign up using Email and Password
Post as a guest
Required, but never shown
StackExchange.ready(
function () {
StackExchange.openid.initPostLogin('.new-post-login', 'https%3a%2f%2fstats.stackexchange.com%2fquestions%2f389230%2fcan-discrete-time-series-be-non-stationary%23new-answer', 'question_page');
}
);
Post as a guest
Required, but never shown
Sign up or log in
StackExchange.ready(function () {
StackExchange.helpers.onClickDraftSave('#login-link');
});
Sign up using Google
Sign up using Facebook
Sign up using Email and Password
Post as a guest
Required, but never shown
Sign up or log in
StackExchange.ready(function () {
StackExchange.helpers.onClickDraftSave('#login-link');
});
Sign up using Google
Sign up using Facebook
Sign up using Email and Password
Post as a guest
Required, but never shown
Sign up or log in
StackExchange.ready(function () {
StackExchange.helpers.onClickDraftSave('#login-link');
});
Sign up using Google
Sign up using Facebook
Sign up using Email and Password
Sign up using Google
Sign up using Facebook
Sign up using Email and Password
Post as a guest
Required, but never shown
Required, but never shown
Required, but never shown
Required, but never shown
Required, but never shown
Required, but never shown
Required, but never shown
Required, but never shown
Required, but never shown
gSnINrOJ82a5IYDL36lKvgLkBZK8J,EUIb9my0ohWNvBJZ2I53f jybbzybS
$begingroup$
You are confused about the meaning of "continuous". Your data is not continuous, it is discrete: the algorithm that draws a line is filling the gaps and displays a continuous line, but the underlying data is discrete. Continuous data exists only in our minds. The world is discrete. Even if the gaps between data points is 1e-100 second.
$endgroup$
– PatrickT
Jan 26 at 10:51